Machine Learning vs Deep Learning : Ultimate Guide 2023
What is machine learning, exactly? operate?
Machine Learning vs Deep Learning Artificial intelligence (AI) known as machine learning (ML) enables software programs to become more accurate predictors without being taught to do so. The past data is used as input by the machine learning algorithms to forecast the value of the output in the future.A very common tool that makes advantage of machine learning is the recommendation engine. Other common uses include prescriptive maintenance, fraud protection, security spam, malware threat detection, and business process automation (BPA).
Why is machine learning so important?
Machine learning is important because it enables businesses to identify trends in customer behavior and operational patterns within their organizations, as well as supports the creation of novel solutions. Many of the top businesses today, including Facebook, Google, and Uber, have made machine learning a crucial part of their operations. For many businesses, machine learning is now a significant competitive edgeWhat are the many kinds of machine learning
Traditional machine learning is often categorized by how an algorithm enhances itself to increase the precision of its predictions. reinforcement learning and semi-supervised learning. The sort of data that data scientists want to forecast will determine the type of algorithm they utilize.
An extensive manual for using machine learning at work
Machine Learning vs Deep Learning Learn more about the numerous applications of AI in business. 10 of the most common commercial applications for machine learning
There are six ways to reduce the various biases in machine learning.
Learn the entire manual for nothing!
About this sort of machine learning, supervised learning, data scientists develop algorithms that incorporate labels for the data they are training. They specify the variables that they want the program to examine to find connections. The algorithm’s input and output are both identified.
Machine Learning vs Deep Learning .Unsupervised learning is a branch of machine learning that relies on algorithms created with unlabeled data. The program searches through the data for any links that can be deemed significant. The predictions or recommendations that algorithms create are predefined, just like the data that algorithms need to build their algorithms.
Semi-supervised training is a machine learning technique that combines the preceding two categories. While data scientists can feed an algorithm with labeled data to train it, the algorithm can also independently study the data and develop its understanding of the information gathered.
Reinforcement learning is a technique that data scientists typically use to train a machine to carry out a multi-step, clearly defined routine. Data scientists design algorithms to complete a task, and then they provide it with either negative or positive signals to help it decide what steps are required to finish the work. The algorithm typically makes its own decisions regarding the steps it should perform along the journey.
How does machine learning work? work under supervision?
Machine Learning vs Deep Learning Using labels on the inputs and the desired results, data scientists must develop the algorithm for a machine that is under supervision. The following are some applications for supervised learning algorithms. dividing data into two categories using binary classification.
The system of classification for several categories: choose from among more than two categories of responses.
Predicting continuous values using regression modeling.
Assembly: combining the outputs of various machine learning models to provide precise predictions.
What is the goal of unsupervised machine learning
Machine learning algorithms that are unsupervised don’t need labels to apply to the data. They examine unlabeled data and search for patterns that can be utilized to divide it into several subsets. Unsupervised techniques are used by the majority of deep learning systems, including neural networks. The unsupervised learning algorithms do well in the following areas:
Clustering Based on similarity, the database is separated into categories.
Analyzing anomalies is the method of finding out-of-the-ordinary data points in a set of data.
Discovering the components of the same data set that are frequently encountered within the exact through association mining. reduction in size minimizing the variety of variables present in a batch of data.
How might one employ semi-supervised learning?
Machine Learning vs Deep Learning When researchers provide a small amount of labeled training data to an algorithm, semi-supervised learning takes place. The algorithm gains knowledge of the dimensions of the data set as a result, which it subsequently applies to unlabeled data. When algorithms are trained on labelled datasets, their efficiency typically increases. Data labelling, however, can be expensive and time-consuming. Semi-supervised learning is a point in between learning that is supervised and learning that is unsupervised in terms of effectiveness. The following are some applications for semi-supervised learning:
Machine translation: Teaching algorithms to translate languages based on an incomplete word list.
Finding fraud-related cases even when you just have a few positive examples is part of the process of detecting fraud.
Data on labelling: Algorithms can learn to automatically apply labels for data to bigger data sets after being trained on small data sets.
Reinforcement learning: what is it?
Machine Learning vs Deep Learning Reinforcement learning works by developing an algorithm with a predetermined goal and a set of rules to achieve it. The algorithm is also programmed by data scientists to look for rewards when it performs actions that move it closer to the objective and to avoid penalties when it takes actions that move it farther away from the goal. It’s used in places like:
Robotics Robots are capable of carrying out tasks in the real world using this technique. Machine Learning vs Deep Learning
Gaming on video: Reinforcement learning has been used to teach bots to play a range of video games.
resource administration Reinforcement learning can help businesses determine the optimal approach to spend resources when there are limited resources and a specific goal in mind. motorcycle accident attorney, motorcycle accident attorneys, motorcycle attorneys, motorcycle accident lawyers, russ brown motorcycle attorneys, attorney, motorcycle accident, georgia motorcycle accident attorney, motorcycle accident lawyer california, personal injury attorney, motorcycle accident lawyer ,motorcycle accident lawyers california, motorcycle, atlanta Cheapest car insurance, best car insurance for you, comprehensive car insurance, third-party insurance, car insurance price, scams involving car insurance, and car insurance rates. Mesothelioma lawyers, mesothelioma lawyer,lawyer mesothelioma, Texas mesothelioma attorney, Texas asbestos lawyer, Texas mesothelioma attorneys, Michigan mesothelioma lawyer, Texas mesothelioma lawyers, Mesothelioma attorneys, Mesothelioma law firm, Texas mesothelioma lawyer, Mesothelioma Houston car accident lawyer, Houston car accident lawyers, Houston car accident attorney, Houston car accident lawyer houston ,attorney ,truck accident attorney, Houston car accident lawyer houston texas, Houston truck accident lawyer, best Houston car accident lawyer, Houston car accident attorneys, Best Houston car accident. Machine Learning vs Deep Learning
Read Also; MTTAMIL
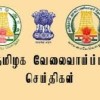